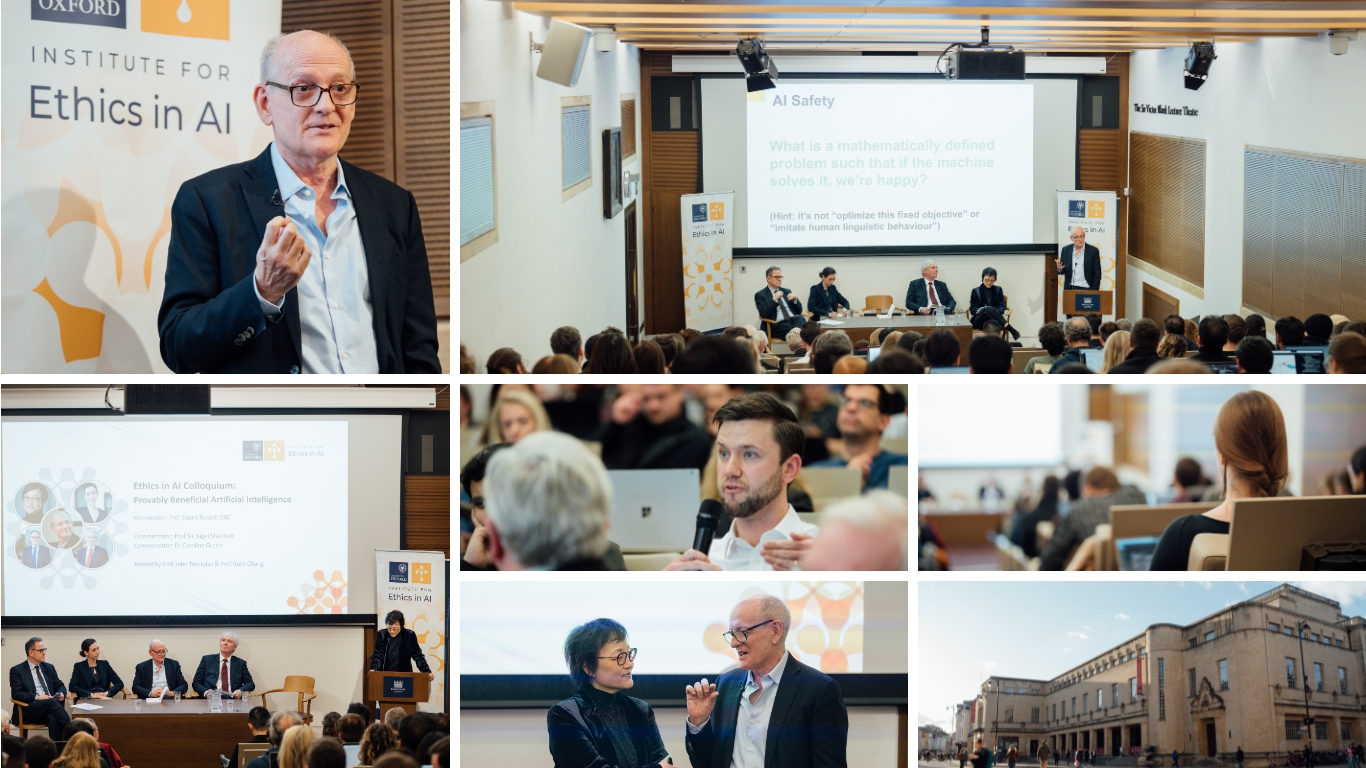
Write-up by Michael Cheng, Doctoral Candidate in Philosophy at the Institute for Ethics in AI.
On 27 February 2025, the Institute welcomed Professor Stuart Russell OBE, Professor of Computer Science at the University of California at Berkeley and author of “Artificial Intelligence: A Modern Approach,” a canonical textbook in AI, at the Ethics in AI Colloquium - Provably Beneficial Artificial Intelligence.
Prof Russell provided his insights on AI development and explored the concept of “provably beneficial AI,” AI systems whose actions can be bounded and controlled using mathematical guarantees.
Russell began his talk by summarising the history of AI as a field, starting with AI’s emergence in the twentieth century as the dream of making intelligent machines to the extent that the machine’s actions can be expected to achieve its objectives. According to Russell, artificial general intelligence (AGI) is AI that can quickly learn to perform as well as or better than a human being in any task in any environment. Although many experts hypothesize that AGI will be achieved within the next decade, Professor Russell was more sceptical: “this is not true.” Russell argued that transformers and current AI technologies are only a piece of the puzzle for building AGI, but they have many limitations. For instance, existing transformers produce outputs in response to inputs, but they cannot sit and think about what to say if you ask them a hard question or an easy question. Moreover, the amount of training data that is being used to train GPTs is exponentially increasing, but that trend cannot continue forever. After all, there is not much more high-quality text left in the universe.
Nevertheless, Russell was optimistic that AGI would be beneficial for humanity if it is developed. According to Russell, AGI could increase world GDP tenfold (by delivering the highest standards of living currently experienced in the world to everyone), provide effective, individualized, and inexpensive healthcare, enable personalized education from brilliant AI tutors, and catalyse much faster scientific research progress. Russell hypothesized that AGI could take us into a “Wall-E world” where AGI is doing everything to run the essentials of civilization for humans, and most humans live reasonably desirable lives. However, this is contingent on our ability to control and design safe AI systems. Intelligence gives humans power over other species in the world, but if we make something more intelligent than us, will humans really retain power over more intelligent entities? Furthermore, there is a “King Midas” problem in that if humans specify an objective for a machine to achieve, the machine might achieve that objective in undesirable ways (e.g., if humans ask for gold, AGI might turn everything into gold).
According to Russell, one solution is to drop the assumption that we specify a fixed objective for AI up front, and instead program AI systems to act in the best interests of humans, but to know that they don’t know what those best interests are. Instead of having humans write down what the objective should be, it’s up to an AI system to gradually figure it out. Russell turned this simple idea into an “assistance game” in the language of game theory, where there is at least one human and one machine who are participants of a game. The human has a payoff function, and the machine’s payoff function is the same as the humans, but the machine does not know what it is. This game can be mathematically solved: the machine defers to the human on what to do half the time, asks questions, and asks permission to do things that will change the world in ways that have any probability of being undesirable to the human. The machine can even be switched off if that is called for by the human’s payoff function, which is also the machine’s payoff function. By programming the machine to have an uncertain objective, the machine will defer to the human’s judgment since the human knows more about the desirability of certain actions. The machine’s actions can be guided by the fact that the machine knows it can be switched off by the human; if the machine makes a choice and is not switched off, then the machine learns something about the underlying payoff function and the machine can learn to match human preferences over time.
Russell argued that this game theoretic example demonstrates that it is possible to derive mathematical guarantees that might someday underpin “provably beneficial AI” that does not deviate unacceptably from human preferences and interests. Moreover, Russell raised several concerns with current AI research approaches. First, many AI researchers implicitly make utilitarian assumptions when programming algorithms, but the way to interpret human behaviour is often contingent on the specific circumstances of how it’s generated, not just aggregating preferences mathematically. Furthermore, should AI systems pay attention to the preferences of the AI designer, the user, everybody, or some weighted combination of preferences? How should the preferences of future generations be weighted? Given that our preferences are often manipulated by others for their own benefit, should AI systems respect those preferences?
Russell concluded by arguing that humans should try very hard to build AI systems that come with some kind of mathematical safety guarantee. Instead of relying on “a giant black box whose internal operations are mysterious but semantically rigorous representations,” Russell proposed that humans should design “provably beneficial AI” grounded by mathematical safety guarantees.