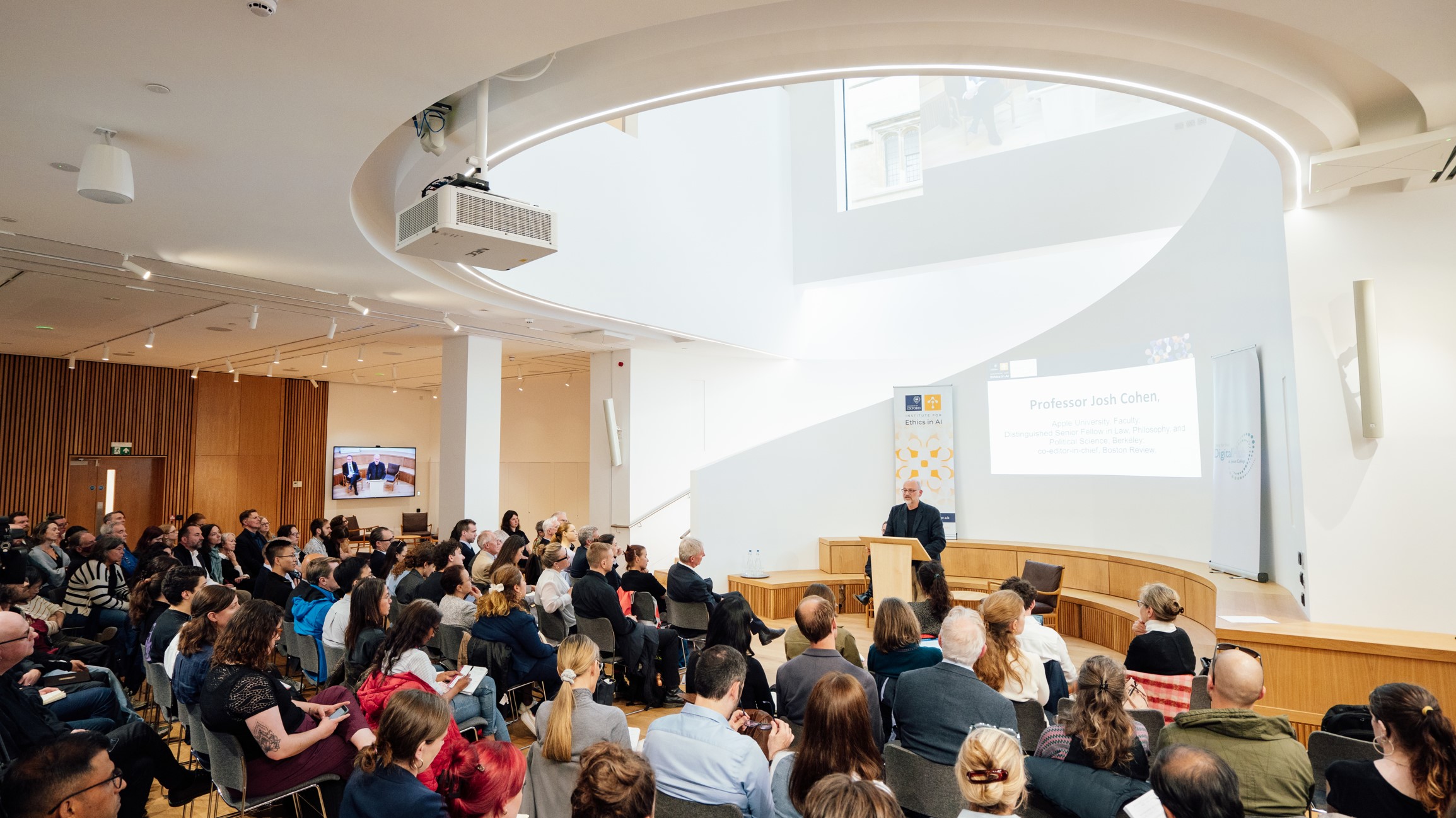
By Helena Ward and Imogen Rivers
On Thursday 6th June we were honoured to welcome Professor Joshua Cohen for the Institute's third Annual Lecture entitled ‘The Reach of Fairness’.
Cohen is a renowned political philosopher who has written extensively on a wide range of topics spanning democratic theory, freedom of expression, religious freedom, distributive justice, political equality, digital technology and global justice. Much of Cohen’s work has been devoted to deepening our understanding of the nature and value of democracy; a focus evident in his reinvigoration of Rawlsian ideas. Having taught at Stanford University and Massachusetts Institute of Technology, he is currently Acting Dean at Apple University and a Distinguished Senior Fellow in Law, Philosophy, and Political Science at Berkeley.
In his lecture, chaired by the Institute’s Inaugural Director, Professor John Tasioulas, Cohen discusses the principle of fairness in machine learning.
Fairness in Machine Learning
The broad principle of fairness has long been a fundamental ideal for ethical, legal and political reflection. In recent years, it has become of paramount importance in discussions concerning the appropriate application of machine learning in the automation of decision-making. This reflects the dramatic uptick in the use of AI to assist in making important decisions: Increasingly, who gets hired, who will benefit from scarce hospital resources and even who gets bail during criminal proceedings will be decided using machine learning algorithms which are trained on vast data sets comprising past decisions. In addition to widespread concerns about the transparency of these algorithms, one of the principal concerns voiced in the academic and public debate is that these algorithms may not be fair. Well-known examples of algorithmic unfairness include recruitment algorithms which systematically reject women as well as facial recognition systems and recidivism predictors which exhibit racial bias favouring lighter-skinned people.
In his lecture, Cohen reminds us that when we’re thinking about algorithmic fairness, we need to start with a view about fairness: what it is, its scope, and reach. In his introductory remarks, Cohen reflects on existing approaches to the analysis and critique of algorithmic fairness and contends that the existing debate has been ‘painting on too small a canvas’. Cohen’s contention is that fairness is a richer and broader value than has so far been appreciated in the academic and public debate on AI ethics. By recasting fairness in a new light, Cohen seeks to reinvigorate normative discourse about machine learning and algorithmic fairness.
Professor Cohen’s talk focussed on the analysis and critique of three ways in which the debate about algorithmic fairness has been truncated.
Three misunderstandings in our current definitions of fairness
- Fairness is not just about group subordination
The first way in which the academic and public debate about algorithmic fairness is overly narrow concerns the excessive focus on what Cohen calls ‘group subordination’. The root of this focus is, in large part, attributable to the standard characterisation of discrimination in terms of the systematic subordination of a group. But Cohen’s first claim is that this doesn't go far enough: there are egregious types of unfair treatment which subordinate individuals without subordinating groups.
For example, Cohen takes us to the context of US employment law and the significance of religious accommodation within that context. But he argues that this emphasis on religious accommodation cannot be understood in terms of group subordination. As he states, ‘claimants ask for religious accommodation as bearers of a conviction, not as members of a disadvantaged group, even less as members of a systematically disadvantaged group’. Cohen’s contention is that what is truly unfair in failures of religious accommodation is the subjection to a 'cruel choice’, that is, forcing a person to choose between their deeply held conviction and their job.
Drawing on Joseph Fishkin's illuminating work on “bottlenecks” in access to employment opportunities, Cohen argues that there are indeed a vast range of legal protections in the employment law context which are not plausibly construed in terms of group subordination. Among the examples touched upon are legal protections against being asked on job applications about current employment status, credit history or criminal convictions. Although these legal protections might indirectly impact group subordination, Cohen argues that it would be inappropriate to characterise them purely in those terms. The idea, on the contrary, is to directly target barriers in access to employment opportunities that are not, or at least not primarily, about systematic group disparities.
What might a fair employment algorithm look like under this expanded concept of fairness? Cohen argues that getting past the focus on group subordination means getting past bottlenecks in access to employment opportunities. We still have reason to adjust an algorithm which screens out applicants on the basis of some characteristic which in effect subjects applicants to a cruel choice or another form of bottleneck. This is so, Cohen contends, even if this generates no systematic group subordination.
- A focus on fair organisational decisions has limited utility in advancing equality of opportunity.
Much of the current work on fairness focuses on the risk of fairness in organisational (often private) decision-making. In doing so, fairness has been understood and evaluated within a restricted domain. Cohen’s second claim is that the view of fairness adopted in organisational decision-making provides a fundamentally limited approach to advancing equality of opportunity: it is too fine-grained, or narrow.
Views of fairness used to assess algorithmic decision-making tend only to focus on organisational decisions, not on the background social structures relating to those decisions, such as laws and public policies. Within this restricted domain, it is much harder to advance the goal of equality of opportunity.
Take an algorithm which allocates kidneys for transplant. There are more individuals on the allocation list than there are transplants a year, so we need some way of achieving a fair balance of the different claims of people - a way of allocating transplants fairly. The issue is that whether or not the algorithm decisions are assessed as fair, turns on the scope of the view of fairness we adopt. We could consider whether fairness obtains relative to the people on the list; whether the allocation of kidneys is fair relative to those who need them; whether individuals have fair access to healthcare; or most broadly whether individuals have fair access to health. Organisational decisions, tend to consider fairness within the limited scope of the algorithm's decision-making - decisions which are made relative to the people on the list. But fairness to people on the list is not fairness in allocating kidneys, for there are individuals who have an equal claim to transplants who are not on the list, whether because of a lack of education, or lack of financial resources. And individuals who are not on the list tend to be non-white, and from lower-socio-economic backgrounds.
If we assume that equality of opportunity is a forward-looking requirement: one concerned with creating a fairer society in which the availability of opportunities are freed from the accidents of birth: a world in which where you start does not determine where you land, then narrow views of fairness fall short. Algorithmic fairness of people to the list goes no distance to correcting the unfairness of who gets onto the list. It is not enough, then, just to focus on fairness within organisational decisions - we must also turn to the broader ways in which disadvantages are perpetuated within society more broadly.
In sum, fairness and organizational decision-making provide us with a fundamentally limited approach for advancing equality of opportunity because the fair allocation system itself is unable to rectify the background racial and socioeconomic disparities in health and access to health care inequalities that inevitably shape its allocations. “If our aim is to achieve a fair society, in which Genesis is not Destiny, then organisational decisions which are guided by limited domain views of fairness do too little, too late”.
- While equality of opportunity is a requirement of fairness, fairness has a much broader reach.
Many people are attracted by the view that fairness is not just a virtue of persons, but a virtue of our basic social institutions. In that light, Cohen asks us to consider John Rawls’ idea of justice. Drawing on an idea of fair social cooperation, specifically, as Daniel Chandler has it, cooperation among free and equal persons, Rawls argues for a conception of justice that includes equality of opportunity but also requires protection for basic liberties, including liberties associated with democracy and a distribution of resources that maximises advantage for the least advantaged. Cohen's third claim is that one need not agree on all the specifics of Rawls’ account to feel the force of this expanded concept of fairness.
Rawls’ proposal for what fair terms of social cooperation are is captured by his two well-known principles of justice. Firstly, each person has an equal claim to a fully adequate scheme of equal basic liberties, which scheme is compatible with the same scheme for all. Secondly, economic inequalities are to satisfy two conditions. First, they must be attached to positions and offices open to all under conditions of fair equality of opportunity. Second, they are to be of greatest benefit to the least advantaged in society.
Cohen's radical proposal is to focus on this latter principle, the ‘difference principle’, as a concept of fairness to guide the development of AI decision-making. Drawing on cutting-edge empirical research, Cohen considers how the development of large-language models might be used for the benefit of lower-skilled workers. The suggestion is that this might help to counteract the upheaval which advances in AI both promise and threaten for the future of work. Cohen goes on to draw out the radical consequences which a Rawlsian principle of fairness would have on the distribution of income and wealth if we allow it to shape the development of generative AI.
The question sits open at the end of the annual lecture: how else might a tailored development of AI in line with an expanded conception of fairness, such as the Rawlsian difference principle, guide our advancement towards a fairer and more just society?